The use of Artificial Intelligence and Biomarkers is crucial in the diagnosis, prediction, and monitoring of disease.
- Lawrence Cummins
- Jan 1, 2024
- 4 min read
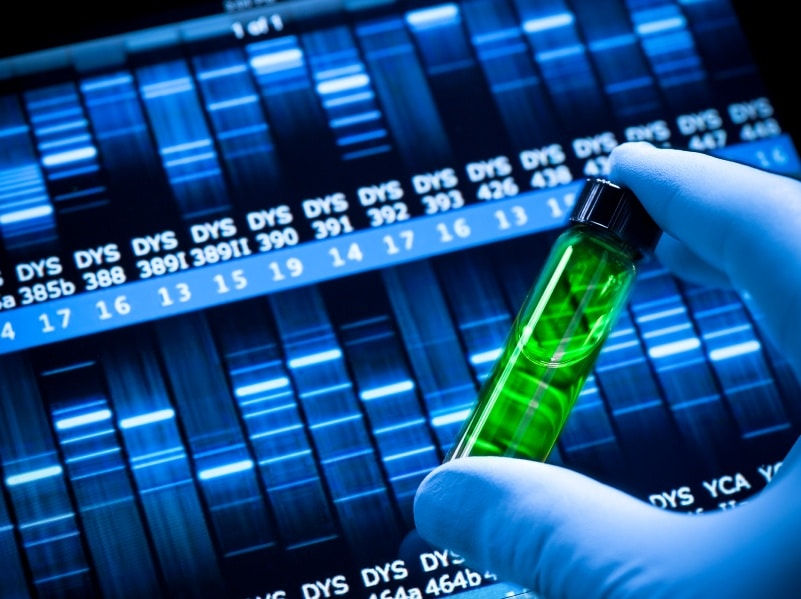
Biomarkers play a crucial role in diagnosing, predicting, and monitoring various diseases and conditions. They can be found in blood, urine, tissue, or other bodily fluids and are used to inform about normal or abnormal biological processes or responses to a therapeutic intervention.
Advancements in technology, particularly in the field of artificial intelligence (AI), have revolutionized the way biomarkers are utilized in clinical practice. AI and other related technologies, such as Artificial Neural Networks (ANN), Convolutional Neural Networks (CNN), and Deep Learning, can significantly enhance the discovery and utilization of biomarkers in various medical settings.
AI can be utilized to identify and analyze biomarkers using large datasets and complex algorithms, leading to the discovery of new biomarkers or the validation of existing ones. Artificial Neural Networks (ANN), Convolutional Neural Networks (CNN), and Deep Learning are subsets of AI that are specifically designed to mimic the way the human brain processes information. These technologies can recognize patterns and make predictions based on vast amounts of data, making them invaluable tools in biomarker research and application.
One of the ways in which AI, Artificial Neural Networks (ANN), Convolutional Neural Networks (CNN), and Deep Learning can be used in biomarkers is through medical imaging analysis. For example, CNN can be trained to analyze MRI or CT scans to detect subtle changes in tissue that could serve as biomarkers for disease progression or treatment response. This can be particularly useful in early cancer detection, where the identification of biomarkers could lead to earlier and more effective interventions.
AI can integrate and analyze diverse data types, such as genetic, proteomic, and metabolomic information, to identify novel biomarkers or develop multi-marker panels that comprehensively understand a patient's health status. The ability of AI to handle large and complex datasets allows for a more thorough exploration of the relationships between different biomarkers and their predictive power for specific diseases.
AI can aid in developing personalized medicine by identifying biomarkers unique to an individual's genetic makeup, lifestyle, and environmental factors. This can lead to tailoring treatments and interventions based on a patient's specific biomarker profile, potentially improving outcomes and reducing adverse effects.
In addition to biomarker discovery, AI can also be used to assess biomarkers' predictive and diagnostic power by constructing predictive models and algorithms. This can help determine the clinical utility of biomarkers and their potential use in risk stratification, disease prognosis, and treatment selection.
As the global population continues to age, the prevalence of age-related diseases such as cancer, obesity, and diabetes is increasing at an alarming rate. The growing number of cancer therapies and diabetes treatments, as well as the longer lifespans of cancer patients, presents a significant challenge for healthcare systems worldwide. In this context, the use of artificial intelligence (AI) with biomarkers has the potential to play a crucial role in addressing these challenges and improving patient outcomes.
One of the most pressing issues facing the healthcare industry today is population aging. With advancements in healthcare and technology, people are living longer than ever before. However, with increased age comes an increased risk of developing chronic diseases such as cancer, diabetes, and obesity. The use of AI with biomarkers can aid in the early detection and prevention of these age-related diseases. By analyzing vast amounts of data from biomarkers, AI can identify patterns and correlations that may indicate the likelihood of developing a specific disease. This can lead to earlier interventions and personalized treatments, ultimately improving the quality of life for elderly individuals.
Moreover, the rising rates of obesity have reached epidemic proportions in many parts of the world. Obesity is a significant risk factor for a range of health issues, including cardiovascular disease, diabetes, and certain types of cancer. AI can analyze biomarkers such as genetic and metabolic markers to identify individuals at high risk of obesity-related complications. By understanding the underlying mechanisms of obesity and its associated diseases, AI can help in the development of more targeted interventions and personalized treatment plans for obese individuals.
In addition, the growing number of cancer therapies and diabetes treatments poses a significant challenge for healthcare providers. With a vast array of treatment options available, it can be challenging to determine the most effective approach for each patient. AI, in combination with biomarker analysis, can help identify the most suitable treatment for an individual based on their unique genetic and biological profile. By leveraging AI algorithms to analyze biomarker data, healthcare providers can make more informed decisions about treatment strategies, leading to improved patient outcomes and reduced healthcare costs.
Technological advancements have greatly improved our ability to analyze the increasing number of biomarkers. High-throughput technologies such as genomics, proteomics, and metabolomics generate vast amounts of data that can overwhelm conventional analytical methods. AI can help extract valuable insights from these large datasets, identify relevant biomarkers, and develop predictive models for disease progression and treatment response. This has the potential to revolutionize how we diagnose, treat, and manage age-related diseases, ultimately leading to better patient outcomes.
Advances in bioinformatics have played a critical role in facilitating the integration of AI with biomarker analysis. Bioinformatics tools and databases enable large-scale biological data storage, retrieval, and analysis, providing a foundation for AI-driven biomarker discovery and validation. This interdisciplinary approach has the potential to yield novel biomarkers for age-related diseases, paving the way for the development of innovative diagnostic and therapeutic solutions.
The use of AI with biomarker analysis has the potential to address the healthcare challenges associated with the aging population, rising rates of obesity, a growing number of cancer therapies, diabetes treatments, and longer lifespans of cancer patients. By leveraging AI algorithms and bioinformatics tools, healthcare providers can unlock the potential of biomarkers to personalize treatments, improve patient outcomes, and alleviate the burden of age-related diseases on healthcare systems worldwide.
Comments